IMS group's new paper on “Fully learnable deep wavelet transform for unsupervised monitoring of high-frequency time series” has just been published in PNAS
The proposed methodology combines the best of two worlds: a thorough theoretical foundation on the wavelet properties with the learning ability of deep learning
The novel fully unsupervised deep-learning framework for high-frequency time series is able to extract meaningful and sparse representation of raw signals and is able to handle different lengths of time series flexibly, overcoming thereby several of the limitations of existing deep-learning approaches.
The proposed architecture could demonstrate a significant improvement on sound data processing, both for classification and for anomaly detection tasks. Our approach allows the use of the raw high-frequency data as input to a deep-learning architecture, a setup usually avoided in the literature due to the difficulty of designing efficient architectures that are robust to changes in the input lengths.
The proposed architecture takes root in spectral analysis and can replace the usual preprocessing steps such as spectrogram or wavelet coefficient extraction. Since it is unsupervised, it can be used as an input to subsequent learning methods. In addition, compared to other deep-learning architectures, it is a very light framework with only a few hundred learnable parameters, mitigating in that way the high risk of overfitting. With its spectral interpretation, it also provides diagnostics information to the domain experts that can potentially improve the interpretation capabilities.
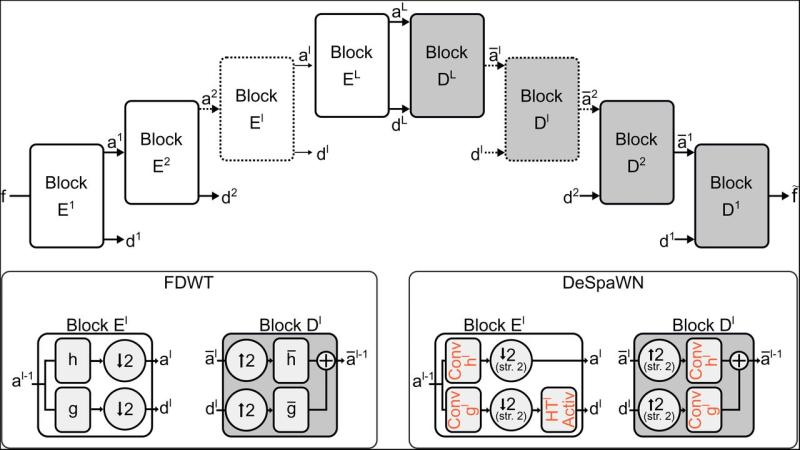