New paper entitled "Real-time model calibration with deep reinforcement learning" has been published in the Mechanical Systems and Signal Processing journal
A common challenge in physics-based modeling of complex systems is the inference of model parameters from observations. In our recent publication, 'Real-Time Model Calibration with Deep Reinforcement Learning,' we propose how reinforcement learning enables real-time inference of model parameters on complex systems such as turbofan engines.
Our RL-based approach does not need ground truth model parameters for training, and our experiments show that the proposed approach generalizes better than neural networks based on supervised learning. Also, the proposed RL-based approach is faster than Kalman Filter estimations while providing a more stable inference of model parameters under the condition of noisy measurements and uncertainty on the underlying physics-based model.
Check out our external page paper for more details!
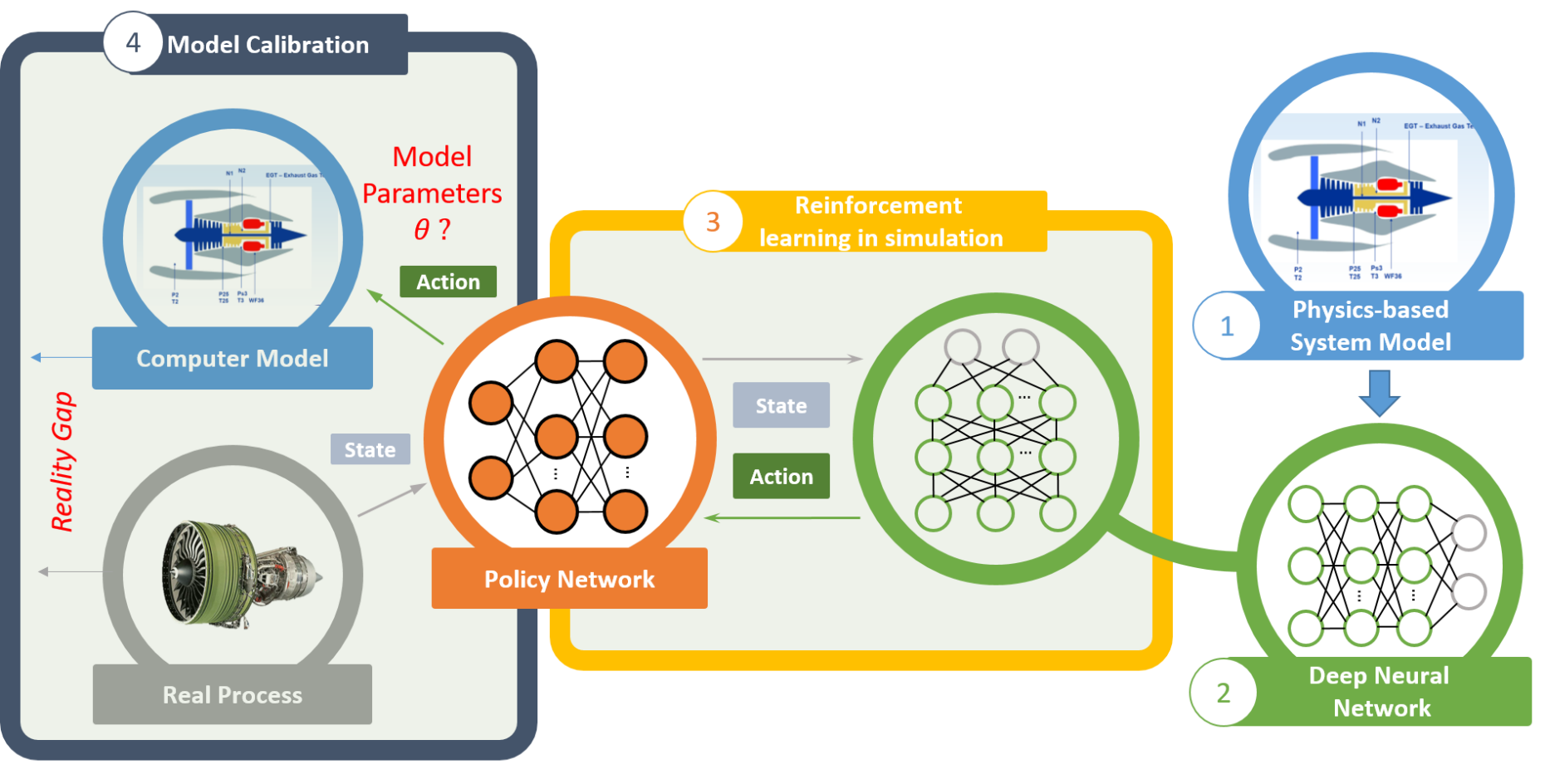
The Chair of Intelligent Maintenance Systems focuses on developing intelligent algorithms to improve performance, reliability and availability of complex industrial assets and making the maintenance more cost efficient. For more information visit our webpage