09
Our new paper "Uncertainty-Aware Prognosis via Deep Gaussian Process", has been published on IEEE Access
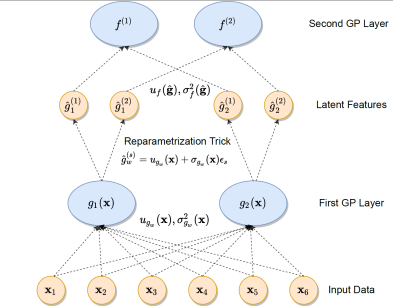
Uncertainty Quantification (UQ) is key in applications where erroneous decisions due to overly confident predictions can lead to catastrophic consequences. An example of such applications is when machine learning algorithms are utilized to predict the remaining useful life (RUL) associated with a certain industrial asset.
Our work on Contrastive Feature Learning for Fault Detection and Diagnostics has been presented at the 31st European Safety and Reliability Conference (ESREL)
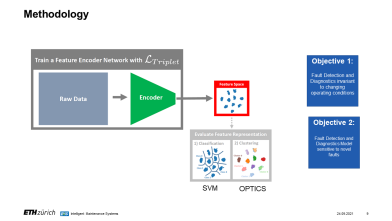
The hybrid conference in Angers again emphasized on the great potential and advances of using AI in the context of safety related domains such as health monitoring, diagnosis and maintenance in general.
Algorithms for experience transfer between experts and novices for the operation of complex systems on the example of Utility Tunnel Boring Machines

Optimal operation of complex systems requires substantial experience by skilled operators which may take long to acquire. Such operational experience may be difficult to formalize and consequently to transfer to novices. The importance and difficulty of such transfer of experience is particularly paramount for systems operated under high uncertainty and high requirements on operational performance, such as for example, for tunnel boring machines.
New PhD student Ismail Nejjar joins the IMS Group
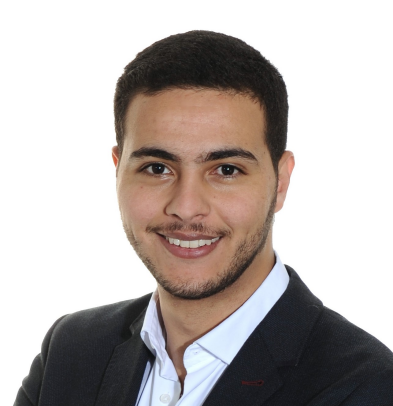
Ismail Nejjar holds degrees in Robotics with a focus in Deep Learning (MSc) and Microengineering (BSc) from the Swiss Federal Institute of Technology in Lausanne (EPFL).
New paper entitled "Real-time model calibration with deep reinforcement learning" has been published in the Mechanical Systems and Signal Processing journal
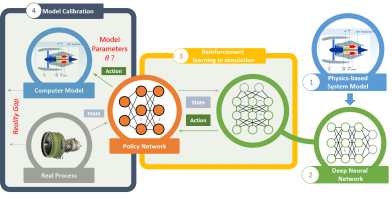
A common challenge in physics-based modeling of complex systems is the inference of model parameters from observations. In our recent publication, 'Real-Time Model Calibration with Deep Reinforcement Learning,' we propose how reinforcement learning enables real-time inference of model parameters on complex systems such as turbofan engines.