Our new paper "Uncertainty-Aware Prognosis via Deep Gaussian Process", has been published on IEEE Access
Uncertainty Quantification (UQ) is key in applications where erroneous decisions due to overly confident predictions can lead to catastrophic consequences. An example of such applications is when machine learning algorithms are utilized to predict the remaining useful life (RUL) associated with a certain industrial asset.
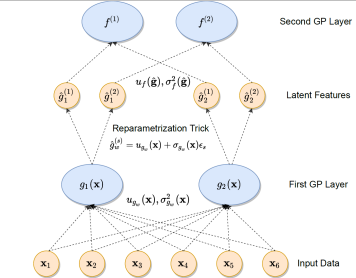
Typically, these techniques are given high dimensional sensor data as input and output an estimate of the RUL in the form of a point estimate. This approach might result in completely wrong predictions when the input data are noisy or when there's a high level of intrinsic uncertainty in the data. To tackle this issue, we evaluate the performance of different types of Deep Gaussian Processes to predict a distribution over RUL values, in such a way that uncertainty can be quantified. Deep Gaussian Processes enjoy the benefits carried by both Deep Learning methods and Gaussian Processes, in light of their high level of expressivity and their ability to perform UQ.
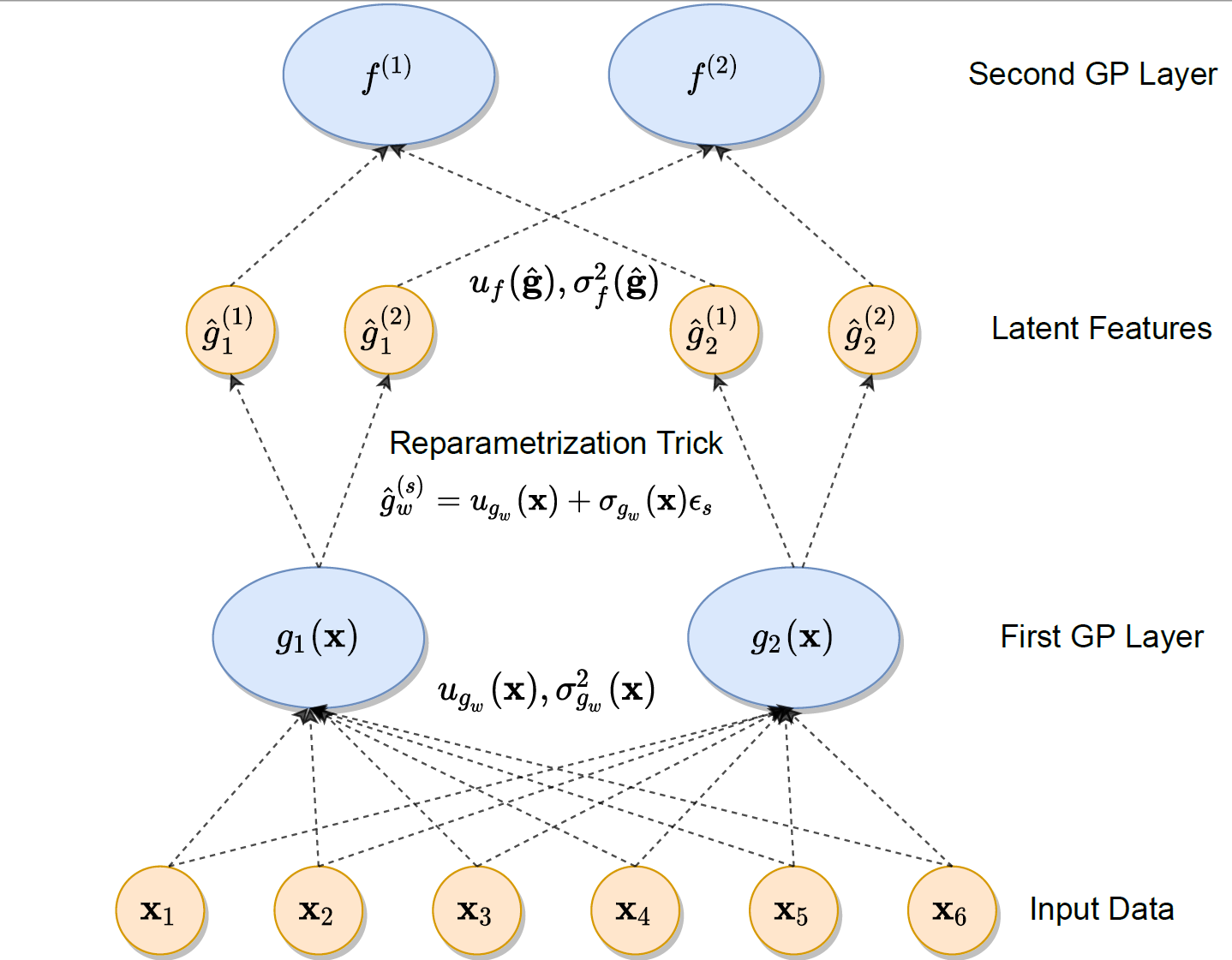