Our work on Contrastive Feature Learning for Fault Detection and Diagnostics has been presented at the 31st European Safety and Reliability Conference (ESREL)
The hybrid conference in Angers again emphasized on the great potential and advances of using AI in the context of safety related domains such as health monitoring, diagnosis and maintenance in general.
The four day conference provided insight in high level concepts such as the digital twin, insights in specific application from various industrial sectors (railway, off-shore systems, etc. ) including the specific and general challenges as well as new methodological development to conquer these.
In this context, we have presented our work that tackles the challenge of developing a diagnostics model that is on the one hand robust to changing operation conditions (shift in the data distribution) at inference time while still being sensitive to novel fault patterns (anomaly detection). To achieve that, a suitable feature representation is trained with a contrastive objective, that is classified (to classify known faults under changing operating conditions) and clustered (to detect anomalies) in a subsequent step.
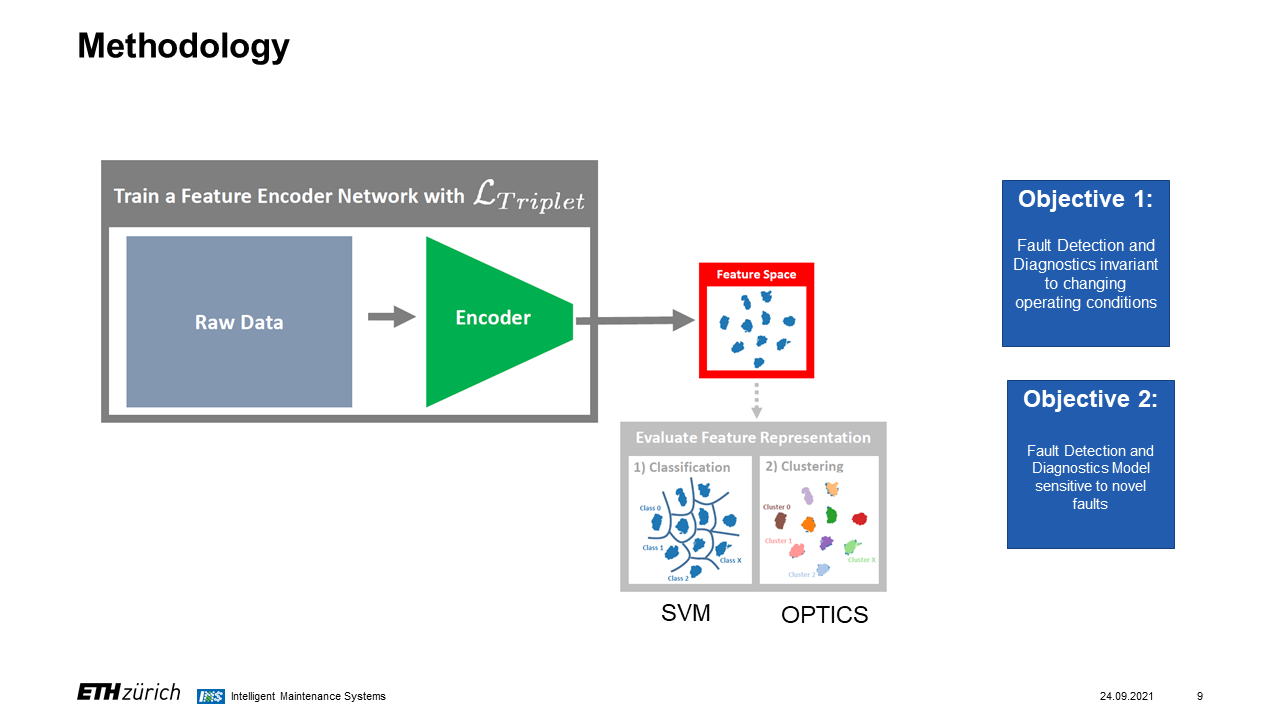
The Chair of Intelligent Maintenance Systems focuses on developing intelligent algorithms to improve performance, reliability and availability of complex industrial assets and making the maintenance more cost efficient. For more information visit our webpage